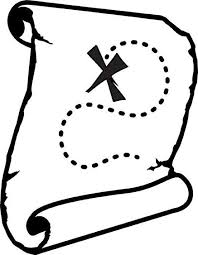
Experimentation is routinely used to develop vaccines that save lives, improve professional sports, and develop new consumer products. It is also widely used to test and improve public policy. Indeed, in 2019, the Royal Swedish Academy of Sciences awarded the Nobel Prize in economics to a trio of development economists, because “their experimental approach to alleviating global poverty” has “dramatically improved our ability to fight poverty.”
And yet, experimentation is largely absent from one field where you would likely expect to find it most: technology policy.
The basic idea isn’t revolutionary: Use a trial to gather data on what works and what doesn’t, and then use that data to make products better and rules smarter. This approach is used in fields where the stakes are high (medicine), where people have strong allegiances (sports), and where novel theories are being tested (economics). Yet in technology policy, experimentation is anathema.
This is troubling. Lack of technology policy experimentation puts the United States at a strategic disadvantage globally, as other countries have been willing to experiment with new regimes in technology policy. It also limits U.S. domestic policy because the lack of experimentation makes it more difficult to pass sensible reforms to improve the U.S. tech sector. Instead of implementing policy tools that will help regulators to learn and then improve policy incrementally over time, tech policy in America is at a standstill.
The United States Needs Tech Policy Experiments
Experimentation is particularly well-suited to policy development in fields like technology, where the impact of rules on products and markets is so uncertain and the consequences of reform seem immense. Will privacy rules enhance competition or entrench Big Tech? Do speech constraints reduce misinformation or increase it? Will merger restrictions enhance or limit the ability of start-ups to compete?
When people debate these sorts of questions, each side digs in its heels. Proponents of reform suggest that changes will be cost free. A better tomorrow is possible, they argue, without any trade-offs. Opponents suggest that changes will be catastrophic, upending the internet as we know it. If the rules change, then no product will work, no person will be secure, and no information will be private.
Of course, any new tech policy will produce both costs and benefits. The challenge is to identify them in advance. Because regulators lack concrete information about the impact of a policy proposal, they struggle to craft policy that balances these trade-offs honestly and transparently. In the absence of good data, policymakers risk passing laws that quickly become unpopular after failing to address the problems they were designed to solve.
Another barrier to tech reform is that the stakes seem too high. Both proponents and opponents of a policy position are left arguing for a policy that could last a lifetime. One side may seek to push through the most ambitious reform it can, knowing that getting legislation through the political process might be the last opportunity for reform for a generation. The other side may view a loss as catastrophic, since defeat might mean that the potential scope of product development is narrowed for a lifetime. This encourages extremism and discourages compromise. The result is that the federal regulatory regime governing the tech sector looks almost the same as it did 20 years ago.
Tech policy should be more nimble and move quickly to take into account new technologies and new understandings of the impact of public policy on people’s lives. Experimentation can help.
A Road Map for Tech Policy Experimentation
States, Congress, and administrative agencies should use experimental design principles to inform policy development. Doing so will result in smarter, stronger, more enduring tech policy. Where to start?
First, experimenters need data. Tech policy has not been designed to generate the kind of data that would allow researchers to study what works and what doesn’t. How well does Virginia’s privacy law protect the privacy of Virginia’s citizens, and what impact does it have on innovation in the state? To answer those types of questions, participants must share sufficient data so that it is possible to understand what worked and what failed.
Data sharing ideally would occur within a broader framework of auditing and review, in which a trusted third party or committee monitors and evaluates the performance of the experiment. This third party might then also be responsible for publishing a report—which might be made public in part or in its entirety—to recommend continuing or ending the trial.
This requires a legal regime that incentivizes data sharing. There is a lot of talk about transparency these days—and there is a push in Congress for tech platforms to share more data with researchers. To make it possible to share data at the scale necessary to evaluate the efficacy of a law, researchers need to be able to access data without fear of retribution and platforms need to be able to share data without worrying that it will open them up to liability for violating users’ privacy. Note that all of the sensible transparency provisions that have been proposed so far—like the Knight Institute’s model safe harbor for platform researchers and the proposed Platform Accountability and Transparency Act—have safe harbors for platforms to incentivize them to participate in data sharing. This is essential.
One option to promote data sharing is to encourage self-regulatory organizations like the Digital Trust & Safety Partnership (DTSP) and the Global Network Initiative (GNI) to include more granular data in their assessments of tech company practices. For instance, DTSP might focus one assessment on age-gating techniques to address child safety issues, and ask platforms to provide data on how different product models perform in practice. To help lawmakers design better policies, these reports would need to go beyond the language of press releases—where companies speak in general terms about how effective their tools are—and instead provide detailed information on both the successes and the failures of different product implementations. These reports would surface the type of data that would enable regulators to understand why one specific approach to age-gating worked better than another.
Of course, data sharing alone isn’t enough to ensure that data will inform public policy. Many academic researchers focus their work on academic audiences, so this work is often overlooked by policymakers. To close this gap, foundations should incentivize more policy-relevant academic research, such as by explicitly funding translational work that uses empirical research to shed light on pressing questions in tech policy.
Government could also develop and use its own data to inform the policy process. Currently, the executive branch routinely uses cost-benefit analysis to assess the impact of agency rules, but this process is used much less frequently to assess the potential impact of legislation. (The Congressional Budget Office now scores legislation to assess its impact on the federal budget, but that estimate of cost considers only a sliver of the information in a full-fledged cost-benefit analysis.) Cost-benefit analysis is not intended to offer a dispositive judgment on whether a bill is good or bad. Rather, it helps to provide information that will assist decision-makers in identifying and weighing potential policy trade-offs. Congress should task the Government Accountability Office with developing cost-benefit analyses for high-priority tech policy proposals, and should publish those analyses so that policymakers and the public can debate them. Companies could also publish cost-benefit analyses to shed light on their decisions, similar to the human rights impact assessments they publish to provide insight into how they address human rights risks.
Second, experiments should limit the liability of participants. People and organizations won’t take risks with new approaches if they fear that any attempt at innovation could mean that they face lawsuits or negative publicity. Imagine, for example, what regulators could learn from the self-driving data collected by automakers like Tesla. But what incentive do automakers currently have to share that data when there’s a good chance it would just be used against them? If anything, the incentive is to shield that data from regulators until they are forced to hand it over.
Platforms might be more willing to share granular data if they feel confident that data won’t subsequently be used in a prosecution against them. For example, if the Federal Trade Commission (FTC) were to test new labeling requirements for advertisements, it should provide a safe harbor to the advertising platforms for the duration of the experiment. Tech platforms should be incentivized to participate in designing smarter regulatory oversight, and safe harbors have a long history of doing just that.
One good example of this dynamic is the FTC’s August 11 announcement that it is “exploring rules” on privacy and security, including how company practices impact teenagers. In the context of a rulemaking procedure, companies will likely be hesitant to share detailed information about the safety costs and benefits of various product decisions they make.
Alternatively, government agencies could use their convening power to limit the possibility of liability. The FTC could convene workshops to gather data on best practices for safety interventions—such as which types of parental controls work and which don’t—and commit to use information gathered in the course of those discussions only to inform nonbinding policy guidance, and not for rulemaking or enforcement. If they commit to ensuring that the guidance is nonbinding in law and in practice, companies might be more inclined to share meaningful data.
Third, regulatory experiments should follow the basic rules of all scientific experimental design. This means that, whenever possible, experiments should develop clear hypotheses to be tested; randomly assign treatment conditions (in this case, the new experimental policy); and, if possible, be replicated. Without some controls, it can be hard to identify whether a policy’s outcomes are a result of the policy or are simply coincidences.
Fourth, regulatory experiments should be time and scope limited, and they should be designed for iteration. There is no “beta” version of a bill; there is just the final product. And if a policy produces a bad result, there is no easy way to update it based on feedback data. Even if California’s privacy law were to suppress venture capital investment—and some preliminary evidence on similarly styled laws indicates that it could—there is currently no mechanism for revisiting the law in light of its actual impact.
Experiments test for fixed periods of time and often apply only to fixed population segments or geographic locations. Suppose the FTC is considering guidance regarding the labeling of online advertisements. The FTC could work with advertising platforms like Alphabet, Meta, Snap, and Reddit to run time-limited experiments followed by surveys to learn which labels have the best impact.
The Regulatory Sandbox
Policy experimentation might take many forms, but one encouraging model is the regulatory sandbox. This model has been used worldwide—from Singapore to the United Kingdom—and for issues as diverse as privacy and financial technology. In the United States, the Consumer Financial Protection Bureau implemented a sandbox and granted “no action letters” to several companies to permit them to experiment with novel financial products. It has now also been codified into law in several states, principally as a tool for experimentation with financial technology products.
The sandbox model embodies many of the core components of policy experimentation. A sandbox specifies the boundaries of an experimental trial in terms of eligible products and scope, invites applications from industry to test innovations that meet those terms, incentivizes industry to participate by offering them regulatory relief for tests they conduct within the sandbox, and creates a structure for generating data and reviewing performance. Without the protection of a sandbox, a company would likely be hesitant to conduct large-scale tests that could expose it to costly lawsuits or damning headlines.
Sandboxes could be used in tech policy to explore the interplay between novel products and novel policy. For example, if there is uncertainty about how data portability might impact privacy, a sandbox on data portability could be used to incentivize companies to trial products that dramatically expand a user’s ability to move data between two different services. In the wake of a sandbox trial, legislators would have information that would enable them to craft stronger laws on data portability.
Although sandboxes have generally been favored by the right and viewed skeptically by the left, they could be used to advance the tech policy priorities of each party. Democrats could use sandboxes to test how platforms could moderate violent content more aggressively. They could use the data sharing features of a sandbox to glean more information about how platform interventions could curb gun violence or reduce harm to vulnerable communities like people of color, women, and children.
Republicans could use sandboxes to encourage platforms to test how they might permit users to see content that might otherwise violate certain provisions of their terms, such as prohibitions on spam and misinformation, or content from accounts that might otherwise be removed from a service.
Each party might try to use a sandbox to affirm its own political preferences, but that’s more difficult to do if the sandbox is accompanied by a strong monitoring and evaluation program. A politician could try to use a sandbox to make a political point, but she would need to wrestle with the data first.
Platforms would almost certainly refrain from these sorts of experiments in the absence of the legal and public relations protection conferred by a sandbox. With the ability to experiment, they might generate insights that would lead to the creation of better products in the future.
Why Policymakers Rarely Experiment, and How That Could Change
If experimentation is so great, why don’t tech policymakers do it more?
One explanation for the United States’ current lack of national experimentation is that policymakers simply do not have enough incentive to take the risks associated with trial and error. Politicians don’t want to be on the wrong side of a failed experiment. It’s also possible that the idea of testing public policy in sensitive areas like data privacy, online expression, and competition may seem reckless or unethical to some. Also, data-driven approaches to public policy have never been known to produce strong results at the ballot box.
These sensitivities are important, and any policy experiment should wrestle with the potential ethical implications of a trial. But those types of concerns have not yet stopped experimentation in areas like medicine, where life and death are in the balance. And if smart tech policy is essential to human welfare, then the harms that come from inaction ought to be just as troubling as the harms that come from experimentation.
Competition with other countries might also spark the need to experiment more in the United States. Europe has become one of the global leaders in tech regulation, passing sweeping laws governing online competition and expression. Those laws will come into effect over the next couple of years and will likely have a dramatic effect on the global tech sector.
There has already been pressure to implement similar laws in the United States, and the U.S. government has already raised concerns about the impact of the laws on American tech companies. More domestic experimentation in tech policy could serve as an alternative to the policy regimes in Europe and provide helpful data on what parts of their regimes are effective and which are not.
Before the United States sets down a new legal regime to govern the digital world, policymakers must make sure that it will improve users’ welfare and lead to a more competitive tech ecosystem. To be more certain about the right tech policy road map for the future, policymakers should experiment with experimentation.
More Stories
Will County, Illinois 1864 Map – May 20, 2023 at 04:14AM
This kid on Google Map trying to get by – April 27, 2023 at 05:05PM
World of Hyatt: Complete list of all-inclusive properties in Europe (with map) – April 27, 2023 at 04:57PM